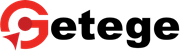
Machine Learning, a term often thrown around in tech circles, but what does it really mean? In simple terms, it's a branch of artificial intelligence (AI) that empowers computers to learn from data and improve their performance without being explicitly programmed. Imagine teaching a child to ride a bike – at first, they stumble, but with each attempt, they get better. That's the essence of machine learning.
Data Input and Processing: At the core of machine learning lies data – lots of it. Machines learn patterns and make predictions based on this data. It's like learning to recognize different breeds of dogs by looking at pictures and characteristics.
Training and Learning: Just like humans, machines need training. Through algorithms, they learn from the provided data. It's akin to teaching a dog new tricks by rewarding desired behavior.
Testing and Improvement: Once trained, the machine is tested with new data to see how well it performs. If it makes mistakes, it goes back to learning, just like how we improve by learning from our mistakes.
Supervised Learning: This is like having a teacher guiding you through each step. The algorithm is trained on labeled data, making predictions or decisions based on that information.
Unsupervised Learning: Here, the algorithm explores data without a teacher. It finds hidden patterns or intrinsic structures in data. It's like discovering new paths in a maze without any hints.
Reinforcement Learning: This is akin to trial and error learning. The algorithm learns by interacting with its environment. Just like a child learns not to touch a hot stove after getting burnt once, reinforcement learning algorithms learn through feedback.
Healthcare: Machine learning assists in diagnosing diseases, personalized treatment plans, and drug discovery, revolutionizing healthcare.
Finance: From fraud detection to algorithmic trading, machine learning is reshaping the financial sector, making processes more efficient and secure.
Marketing and Advertising: Ever wondered how ads seem to know exactly what you need? Machine learning analyzes consumer behavior to personalize marketing strategies.
Autonomous Vehicles: Self-driving cars rely heavily on machine learning to navigate roads, detect obstacles, and make split-second decisions.
Data Quality: Garbage in, garbage out. Machine learning models heavily depend on the quality and quantity of data. Biased or incomplete data can lead to flawed outcomes.
Interpretability: Complex machine learning models can be difficult to interpret. Understanding why a model made a particular decision is crucial, especially in critical applications like healthcare.
Ethical Concerns: With great power comes great responsibility. Machine learning raises ethical concerns regarding privacy, bias, and accountability. It's imperative to address these issues to ensure fair and responsible use.
As technology advances, so does the realm of machine learning. From enhancing personalized experiences to solving complex societal problems, the possibilities are endless. Embracing machine learning responsibly can lead us to a future where machines augment human capabilities, making the world a better place.
Machine learning is not just a buzzword; it's a transformative force shaping our present and future. Understanding its basics can empower individuals to navigate the increasingly digitized world we live in. So, next time you hear about machine learning, remember, it's not magic – it's just smart learning.
Supervised learning is a type of machine learning where the algorithm is trained on labeled data, with input-output pairs, to make predictions or decisions.
Machine learning in healthcare assists in various tasks such as disease diagnosis, personalized treatment plans, drug discovery, and patient monitoring.
Data quality is crucial in machine learning as the accuracy and reliability of the model heavily depend on the quality and quantity of the data used for training.
Yes, machine learning algorithms can exhibit bias, often reflecting the biases present in the data they were trained on, which can lead to unfair or discriminatory outcomes.
Ethical concerns in machine learning include issues such as privacy infringement, biased decision-making, and the potential societal impact of AI-driven automation.