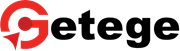
Deep learning is a subset of artificial intelligence (AI) that mimics the workings of the human brain to process data and recognize patterns. It involves training computer algorithms called neural networks to learn from large amounts of labeled data. Imagine it as teaching a child to identify different animals by showing them pictures and telling them what each animal is called.
At its core, deep learning relies on layers of interconnected nodes, much like neurons in the brain. These nodes process information and pass it on to subsequent layers, allowing the network to extract features and make predictions. Through a process called backpropagation, the network adjusts its parameters to minimize errors and improve accuracy over time.
Deep learning has revolutionized various industries, including healthcare, finance, and entertainment. From diagnosing diseases and predicting stock prices to enabling voice assistants and autonomous vehicles, its applications are limitless. It powers recommendation systems on streaming platforms, recognizing faces in photos, and translating languages in real-time.
Neural networks are the building blocks of deep learning models.Neural networks comprise an input layer, intermediate hidden layers, and an output layer.Each layer contains interconnected nodes, or neurons, with associated weights and biases. As data flows through the network, these parameters are adjusted to optimize performance.
Training a deep learning model involves feeding it with vast amounts of labeled data and iteratively adjusting its parameters to minimize errors. This process requires significant computational power and may take hours, days, or even weeks to complete, depending on the complexity of the task and the available resources.
While deep learning has shown remarkable success in various domains, it is not without its challenges. One major limitation is its reliance on large datasets, which may not always be available or representative. Additionally, deep learning models can be prone to biases and overfitting, where they perform well on training data but fail to generalize to new examples.
As technology continues to evolve, so does the field of deep learning. Researchers are constantly pushing the boundaries of what is possible, exploring new architectures, algorithms, and techniques to improve performance and efficiency. The future holds promise for even more groundbreaking applications, from personalized medicine to human-like robots.
With great power comes great responsibility. As deep learning becomes increasingly integrated into our lives, it raises ethical concerns regarding privacy, security, and fairness. Who owns the data used to train these models? How do we ensure transparency and accountability? These are questions that society must grapple with as we navigate the ethical implications of AI.
Accuracy: Deep learning models can achieve state-of-the-art performance in various tasks, surpassing human capabilities in some cases.
Automation: By automating complex tasks, deep learning streamlines processes and increases efficiency across industries.
Innovation: Deep learning drives innovation by enabling new applications and unlocking insights from vast amounts of data.
Deep learning models depend heavily on having access to extensive labeled data for training, which may not always be easily accessible
Computational Resources: Training deep learning models can be computationally intensive and requires high-performance hardware.
Interpretability: Understanding how deep learning models arrive at their decisions can be challenging, raising concerns about transparency and accountability.
In conclusion, deep learning holds immense potential to transform our world, from revolutionizing healthcare to powering autonomous systems. By understanding its principles and applications, we can harness its power for the greater good while addressing ethical concerns and limitations.
1. What makes deep learning different from traditional machine learning?
Deep learning relies on neural networks with multiple layers of interconnected nodes, allowing it to automatically learn representations from data, whereas traditional machine learning often requires manual feature engineering.
2. Can deep learning be applied to any problem domain?
While deep learning has shown remarkable success in various domains, its applicability depends on the availability of labeled data and computational resources.
3. How do deep learning models learn from data?
Deep learning models learn from data by adjusting their parameters through a process called backpropagation, which involves minimizing errors between predicted and actual outcomes.
4. Are there any risks associated with deep learning?
Yes, deep learning models can be prone to biases, overfitting, and ethical concerns regarding privacy and fairness.